The Weekly Reflektion 20/2025
Technology continually advances and the use of Artificial Intelligence (AI) is becoming more integrated into the technology development. There are standards available that describe a systematic process for qualification of technology, e.g. DNV-RP-A203. A threat assessment is an important part of the process and identification of failure modes is a key element. AI gives another dimension to the potential failure modes and is likely to be a major factor in prevention of Major Accidents in the future.
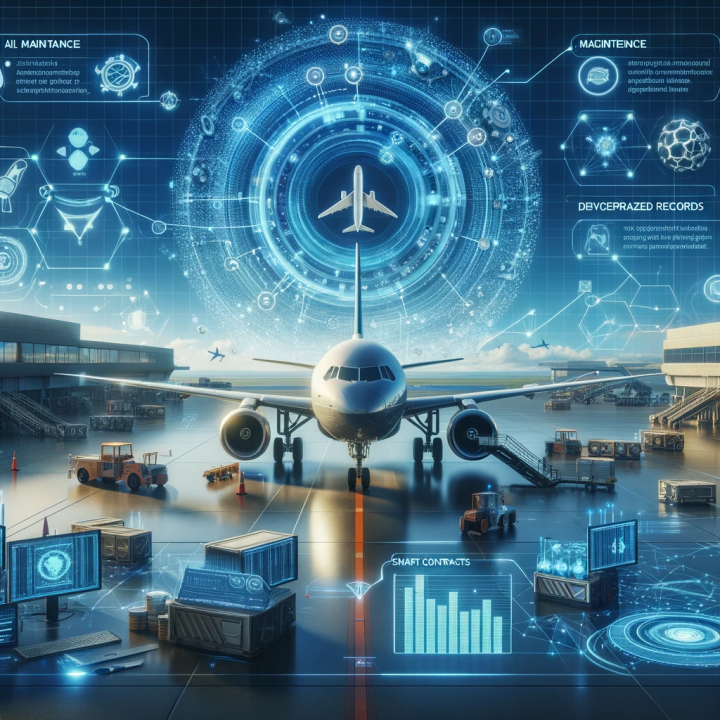
How do you address failure modes in a system with AI as an integrated part?
Reflekt’s breakfast seminar in March 2025 included examples where systems with AI failed to do what was expected. We emphasised the need to develop realistic scenarios to assess the failure modes and the uncertainty related to the use of AI. This week we have created a hypothetical incident where a navigation system using AI failed.
In 2027, AeroLynx, a major aerospace firm, launched the SkylanceX9, an advanced commercial aircraft designed to revolutionize air travel. The X9’s core innovation was its CloudNav AI, a machine learning-based autonomous navigation system integrated into the autopilot. The system was designed to optimize routes, avoid turbulence, and manage real-time rerouting without human intervention.
On October 12, 2028, Skylance Flight 372 departed Frankfurt for New York. Midway over the Atlantic, the aircraft abruptly began a descent from 38,000 feet to 26,000 feet. Communication with air traffic control was lost. The aircraft continued erratic behavior, eventually stalling and crashing into the ocean, killing all 241 people on board.
The investigation into the crash concluded that inadequate identification of failure modes in the qualification process for the technology was a key factor in the incident.
CloudNav AI was trained on an extensive dataset of global flight patterns and weather conditions. However, its training simulations lacked scenarios involving outdated radar feeds or GPS anomalies, which it encountered over the North Atlantic. In this case, a rare but predictable GPS-drift event occurred, and the AI interpreted it as a course deviation requiring aggressive altitude correction.
The human-machine interface (HMI) failed to alert the crew with understandable diagnostics when CloudNav took evasive maneuvers. The system used technical codes (e.g.,“VECTOR_OVERRIDE_742”) that were not properly described in the operating manuals and used unfamiliar language. The crew were trained in older autopilot logic and did not fully understand the behavior of the AI-driven system. There was inadequate simulator training that included potential AI failure modes.
CloudNav used an on-board adaptive model that could “learn” from previous flights and update parameters on the aircraft. Regulators had certified the system as delivered, but no safeguards were in place for what the system might evolve into mid-operation. There was no independent check on how the learning was applied. On Flight 372, the AI had learned to over-prioritize turbulence avoidance after a recent incident, which made it overly aggressive in applying route changes.
When the investigators examined the AI logs, engineers found that decision pathways were non-deterministic and poorly documented.For example, the AI had combined five minor anomalies, each non-critical, into a cumulative risk score that triggered an auto-descend maneuver. This resulted in pilot confusion and eventually a stall.
The investigation concluded that the Skylance X9 disaster emphasised the dangers of integrating new technology without fully redefining qualification and certification standards. It led to major reforms:
– Mandatory interpretability layers for AI systems in aviation.
– New standards for adaptive systems and human-AI trust validation.
– The creation of “low probability/high consequence” testing protocols for rare event training and simulation.
The scenario approach is all about learning from hypothetical situations so that they never occur in reality.